Do You Need to Know Formulas for Quant Cfa if You Use Calcultor
Note: this cheat sheet is updated for the latest 2022'due south curriculum.
CFA exams are tough, we become information technology. We have gone through them ourselves.
That's why nosotros created our Cheat Sheets to aid your review sessions and refresh your retention on important CFA Level one concepts.☕
Each of our Cheat Sheet article focuses on one specific topic surface area for one specific CFA level.
More Cheat Sheets will be published in the coming weeks, sign upward to our member'due south list to be notified beginning.
By referring to the CFA Learning Outcome Statements (LOS), nosotros prioritize and highlight the absolute fundamental concepts and formulas yous need to know for each topic. With some tips at the end too!
Use the Cheat Sheets during your practice sessions to get you to a flying start.
Let'southward dive in – this is a long article, bookmark and come back to it oft 🙂
- CFA Level 1 Quantitative Methods: An Overview
- Reading 1: The Fourth dimension Value of Coin
- Reading 2: Organizing, Visualizing and Describing Information
- Reading 3: Probability Concepts
- Reading 4: Common Probability Distributions
- Reading 5: Sampling and Estimations
- Reading 6: Hypothesis Testing
- Reading 7: Introduction to Linear Regression
- CFA Level 1 Quantitative Methods Tips
CFA Level 1 Quantitative Methods: An Overview
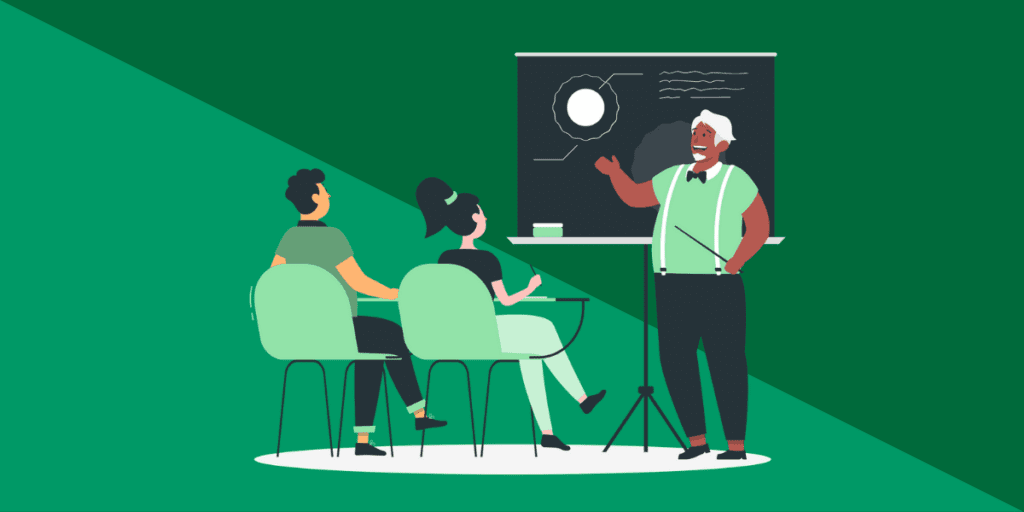
Quantitative Methods is a key foundational topic for CFA Level 1, which forms a basis for Level 2 and Level three learnings.
2022'southward CFA Level 1 Quantitative Methods' topic weighting is viii%-12%, which ways 14-21 questions of the 180 questions of CFA Level i exam is centered effectually this topic.
Information technology is covered in Written report Sessions one and 2, which includes Reading one-seven.
However, this 8%-12% weighting effigy is deceptively low because, as will exist discussed beneath, the cloth covered in this topic area overlaps significantly with textile covered in other areas of the curriculum.
In the context of financial assay, quantitative methods are used to predict outcomes and mensurate results. Our profession seeks to classify majuscule and resources efficiently, and so it is necessary to test hypotheses and quantify whether we are meeting our objectives.
Here's the summary of CFA Quantitative Methods' readings in Level i:
Reading Number | Sub-topic | Description |
---|---|---|
1 | The Time Value of Money | The valuation of assets and securities in different points in time |
ii | Organizing, Visualizing and Describing Data | Various ways of visualising data, measuring cardinal tendencies and dispersion |
iii | Probability Concepts | Using probabilities to predict outcomes when faced with uncertainty |
4 | Mutual Probability Distributions | A give-and-take of normal and non-normal distributions |
v | Sampling and Interpretation | Using a sample to gauge characteristics of a population |
6 | Hypothesis Testing | Determining the level of confidence you lot can have in your conclusions |
7 | Introduction to Linear Regression | Understand the elementary linear regression model and its assumptions, so you can understand the relationship betwixt 2 variables and acquire how to make predictions. |
In the context of financial analysis, quantitative methods are used to predict outcomes and measure out results. Our profession seeks to allocate capital and resources efficiently, so it is necessary to exam hypotheses and quantify whether we are meeting our objectives.
In a nutshell, the CFA Level one Quantitative Methods readings teaches you:
– How to predict the near probable outcomes, or range of outcomes, for future events;
– How confident you can be in those predictions;
– How to calculate the impact of events in one case they take occurred.
Reading i: The Fourth dimension Value of Money
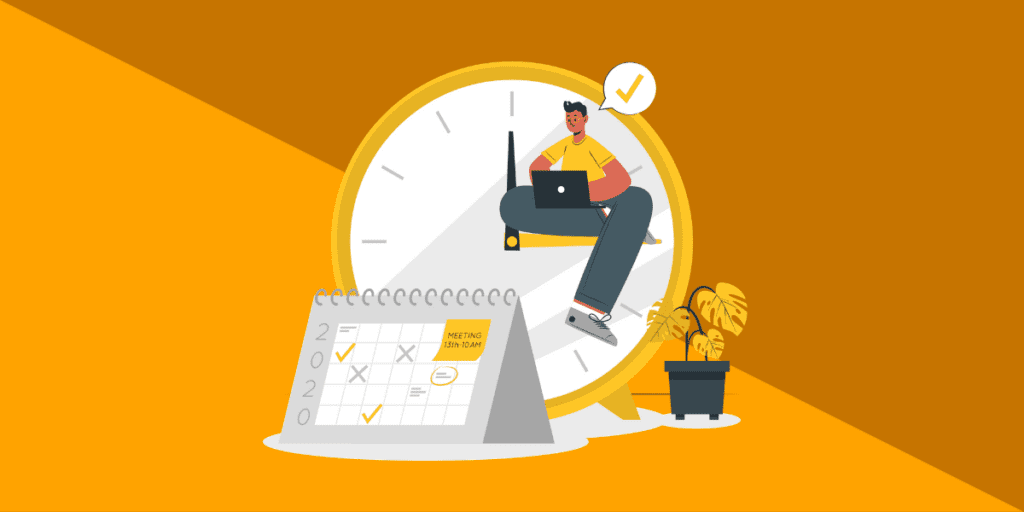
Present Value (PV) and Hereafter Value (FV) of cash flows
\brainstorm{align*} PV&=\frac{FV}{\Large(1+\frac{r}{due north}\Big)^{nt}} \\FV&=PV\Large(1+\frac{r}{n}\Big)^{nt} \end{marshal*}
where r= discount rate, n= number of discounting period per year, t= number of years
Annuity due vs ordinary annuity
Specifically:
- Ordinary annuity = cash menses at the stop-of-fourth dimension menstruation.
- Annuity due = cash flow at the starting time-of-fourth dimension period.
\begin{align*} PV_{annuity \space due}&=PV_{ordinary \space annuity} \times (ane+r) \\FV_{annuity \space due}&=FV_{ordinary \space annuity} \times (1+r) \end{align*}
Perpetuity is just an annuity with space life
And the formula simplifies to:
PV_{perpetuity} = \frac{PMT}{r}
where PMT is the amount of each payment, r is discount rate.
Reading ii: Organizing, Visualizing and Describing Data
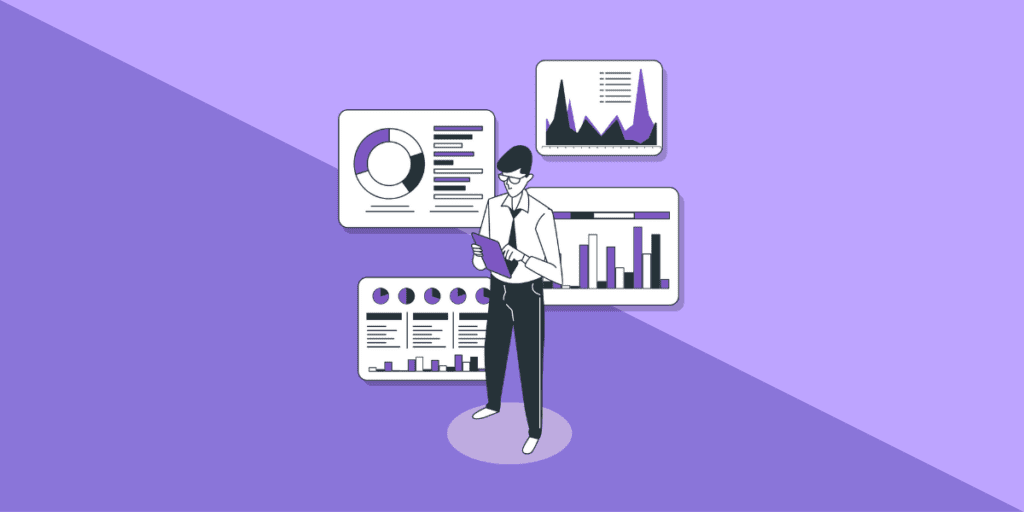
Arithmetic, Geometric and Harmonic Hateful
\brainstorm{align*} Arithmetic \space mean \space(i.e. \space simple \space average)&=\frac{\sum X_i}{N} \\Geometric \space mean&=(X_1 \times X_2 \times ... \space X_n)^{\frac{1}{n}} \\Harmonic \space hateful&=\frac{North}{\displaystyle \sum_{i=1}^{Due north} \Big (\frac{i}{X_i}\Big)} \end{marshal*}
Call back that for the aforementioned dataset:
Arithmetic Mean > Geometric Mean > Harmonic Mean
Population vs sample variance's formulae
\begin{align*} Population \space variance&= \sigma^2 =\frac{\displaystyle \sum_{i=1}^{Due north}(x_i-\mu)^ii}{N} \\Sample \space variance&= s^2 =\frac{\displaystyle \sum_{i=1}^{N}(x_i-\bar{x})^two}{due north-1} \stop{align*}
And the standard deviations for population and sample is just just the foursquare root of the corresponding variance.
Easy right? 🙂
Mean absolute departure (MAD)
Mean \infinite accented \space departure (MAD)=\frac{\displaystyle \sum_{i=ane}^n |x_i-\bar{ten}|}{n-1}
MAD is a measure of the average of the accented values of deviations from the hateful in a data fix. Since the sum of deviations from the mean in a dataset is always 0, we must employ accented values.
Coefficient of variation (CV)
Coefficient of variation (CV) is used to compare the relative dispersion between datasets, every bit it shows how much dispersion exists relative to a mean of a distribution.
CV is calculated by dividing the standard deviation of a distribution with its mean/expected value:
CV=\frac{south}{\overline {X}}
Reading 3: Probability Concepts
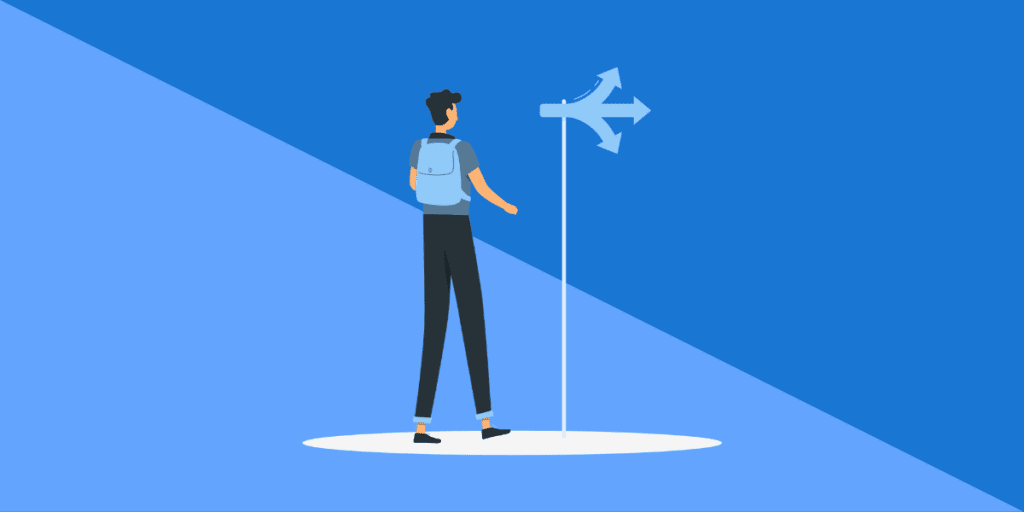
Conditional and joint probability
P(A | B) = P (AB) / P(B)
P(AB) = P(A | B) x P(B). But If A and B are independent, P(AB) = P(A) ten P(B)
P(A or B) = P(A) + P(B)
Expected value of a random variable 10
E(X) = P(101)Xi + P(Xtwo) X2 + … + P(Xnorthward)Xn
Probabilistic variance
\sigma^two(X)=\displaystyle \sum_{i=1}^north P(X_i)[X_i-Due east(Ten)]^two
Correlation and covariance of returns
\rho, corr(R_A,R_B)=\frac{COV(R_A,R_B)}{\sigma (R_A) \sigma(R_B)}
Correlation equals covariance divided by the product of 2 standard deviations.
Expected render on a portfolio
E(R_p)=\displaystyle \sum_{i=ane}^n w_iE(R_i)=w_1E(R_1)+w_2E(R_2)+ ... \space w_nE(R_n)
Variance of a two stock portfolio
Var(R_p)=w_A^2\sigma^two(R_A)+w_B^2\sigma^two(R_B)+2w_Aw_B\rho(R_A,R_B)\sigma(R_A)\sigma(R_B) \\=w_A^ii\sigma^2(R_A)+w_B^2\sigma^2(R_B)+2w_Aw_BCOV(R_A,R_B)
Bayes' Formula
Bayes' formula is basically a method of updating probabilities given new information:
P(Event \space | \infinite Information) = \frac{P(Information \space | \infinite Event)}{P(Information)}\times P(Issue)
Reading iv: Common Probability Distributions
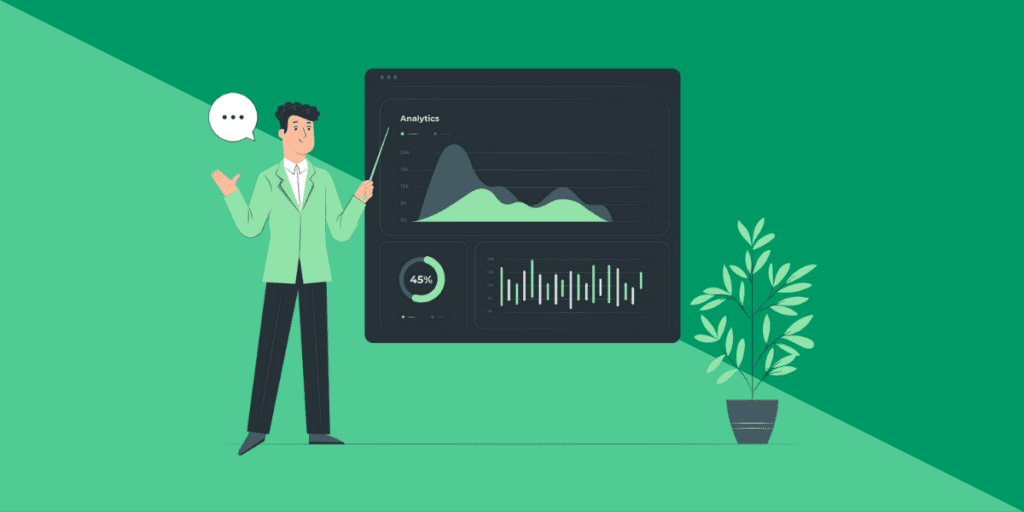
Compatible distribution
Discrete compatible distribution:
P(x)=\frac{1}{n},\infinite where\infinite x=x_1,x_2,...,x_n
Continuous uniform distribution:
F(x)=\frac{10-a}{b-a};\infinite a\le ten \le b
Binomial distribution
The binomial distribution is a sequence of northward Bernoulli trials where the consequence of every trial can be a success (p) or a failure (1-p).
With the probability of success (p) the same for each trial, the probability of x successes in n trials is:
P(X=x)=\space^nC_rp^x(one-p)^n\space^-\space^x
The expected value of a binomial random variable is simply:
Variance of a binomial random variable
\sigma^two=n \times p \times (ane-p)
Normal distribution
The normal distribution is a continuous symmetric probability distribution that is completely described past two parameters: its hateful, μ, and its variance σ2.
- 68% of observations lie in between μ +/- 1σ;
- 90% of observations lie in between μ +/- 1.65σ;
- 95% of observations lie in between μ +/- 1.96σ;
- 99% of observations lie in between μ +/- 2.58σ;
Compute Z-score
Z-score is used to standardize an observation from normal distribution. It shows you the number of standard deviation a given observation is from population mean.
Safety-starting time ratio (SF ratio)
SF ratio is used to measure shortfall adventure:
SF \space ratio = \frac{E(R_p)-R_{target}}{\sigma_P}
Lognormal distribution
The properties of lognormal distributions are:
- It cannot exist negative;
- The upper cease of its range extends to infinity;
- It is positively skewed.
For these reasons, lognormal distribution is suitable for model nugget prices, non asset returns (since returns can be negative).
Pupil's t-distribution
- t-distribution is defined by ane parameter, i.e. degrees of liberty (df) = n-1.
- Information technology is symmetrical, similar bell-shaped distribution to Normal distribution, but has fatter tails and a lower peak.
- Every bit df increases, t-distribution approximates Normal distribution.
Chi-square distribution
- A chi-square test is used to plant whether a hypothesized value of variance is equal to, less than, or greater than the true population variance.
- Chi-square distribution is the sum of squares of independent normal variables. Hence information technology cannot be negative.
- Information technology is asymmetrical and is divers by i parameter, i.e. degrees of freedom (df) = n-ane.
- The shape of curve is dissimilar for each df.
- Every bit the df increase, the chi-square curve approximates Normal distribution.
F-distribution
- F-distribution is a ratio of two Chi-foursquare distributions with two degrees of freedom, hence it cannot be negative either.
- Every bit both the numerator and the denominator's degrees of freedoms increase, the F-distribution approximates Normal distribution.
Monte Carlo simulations
Monte Carlo simulations use computers to generate many random samples to produce a distribution of outcomes. It is used in financial planning, complex derivatives valuation and VAR estimates.
Reading 5: Sampling and Estimations
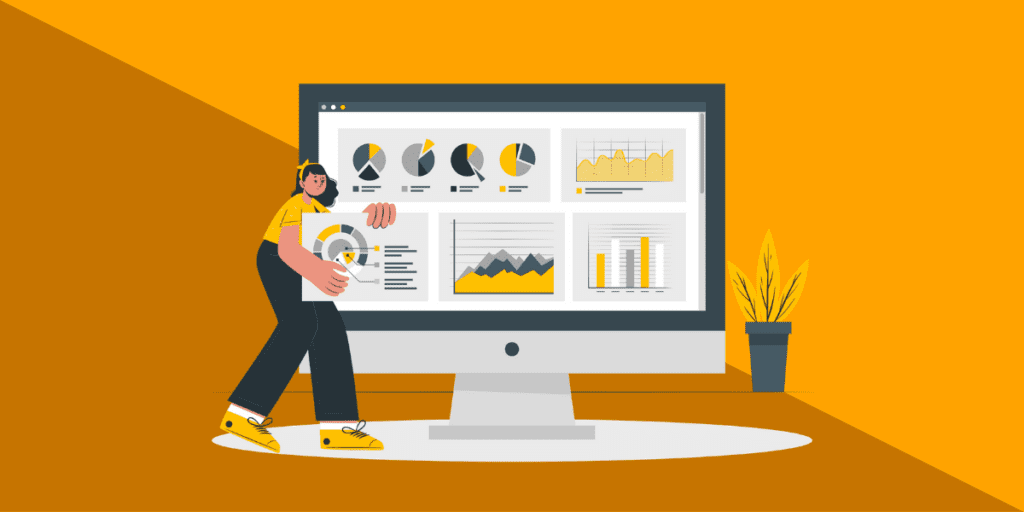
Central Limit Theorem
The key limit theorem states that when we have uncomplicated random samples each of size n from a population with a mean μ and variance σ2, the sample hateful X approximately has a normal distribution with mean μ and variance σii/north every bit north (sample size) becomes large, i.east. greater or equal to xxx.
Standard Error
Standard error of sample mean = standard departure of distribution of the sample means.
If population variance is known, standard error of sample mean is:
\sigma_{\overline{x}}=\frac{\sigma}{\sqrt{n}}
If population variance is unknown, standard error of sample mean is:
s_{\overline{x}}=\frac{s}{\sqrt{north}}
Confidence Intervals
For a given probability, conviction interval provides a range of values the mean value volition be between.
With a known population variance, the conviction interval formula based on z-statistic is:
Conviction \space interval = \overline{X} \space ± \space z_{\alpha/two} \times \frac{\sigma}{\sqrt{n}}
For unknown population variance, the conviction interval formula based on t-statistic is:
Confidence \space interval = \overline{x} \space ± \space t_{\alpha/two} \times \frac{s}{\sqrt{n}}
Reading 6: Hypothesis Testing
vi steps of hypothesis testing
- State the hypothesis (cypher H0 and alternative Ha)
- Identify the advisable test statistic
- Specify significance level
- State conclusion rule
- Collect data and calculate test statistic
- Make a decision
Type I vs. Blazon II error
An area almost candidates oft become dislocated on.
Here are iii unlike ways to nowadays this concept (taken from our article on ways to better study retentivity) which I promise helps your agreement:
- Visually
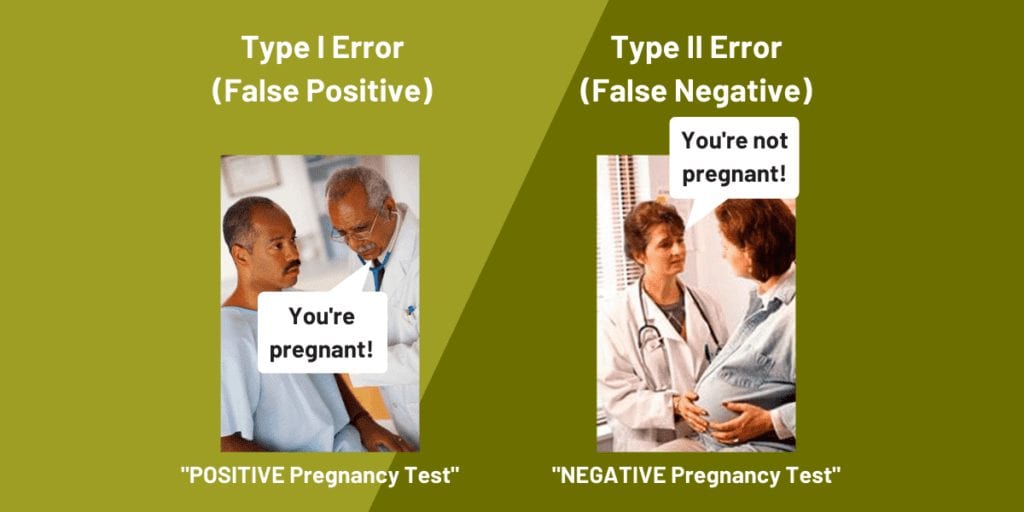
ii) Via a table
H0 is truthful | H0 is false | |
---|---|---|
Turn down H0 | Type 1 Error | Correct rejection |
Fail to Reject H0 | Right conclusion | Type 2 Error |
3) Using messages, for rote memorization if desperate.
OK, you need to have watched Lord of the Rings for this to brand sense, but hither goes:
A Type I error is when you turn down the null when you shouldn't, just like Frodo rejecting the help of Sam, his loyal friend.
A TypeTwo mistake is when you fail to turn down the cipher when you should, just like how Frodo listened to Gollum even though he was a unsafe liar.
To distinguish betwixt them, notation that the 2 ll's in Getllum await like the Roman numeral II, for a Type2 error.
Tests concerning a single mean: when should I utilise z-statistic or t-statistic?
Type of Distribution | Known Variance? | Small sample, n<xxx | Big sample, n>thirty |
---|---|---|---|
Normal | Known ✔︎ | z-statistic | z-statistic |
Normal | Unknown ✘ | t-statistic | t or z-statistic, both are fine |
Non-Normal | Known ✔︎ | – | z-statistic |
Non-Normal | Unknown ✘ | – | t or z-statistic, both are fine |
Tests apropos differences between means (independent samples)
Test statistic is calculated as:
t=\frac{(\overline{X}_1 - \overline{X}_2)-(\mu_1 - \mu_2)}{(\frac{s^2_p}{n_1}+\frac{south^2_p}{n_2})^{ane/2}}
where due south2 p is the pooled estimator of the variance, calculated with this formula
south^2_p=\frac{(n_1-1) s^2_1 + (n_2-1)s^2_2}{n_1+n_2-2}
where the number of degrees of freedom is n1 + nii – 2.
Tests concerning differences betwixt means (dependent samples)
Test statistic is calculated as:
t=\frac{\overline{d} - \mu_{d0}}{s_{\overline{d}}}, \space df= n-1
Test of a single variance (Chi-square examination)
Test statistic is calculated every bit:
\chi^2=\frac{(n-1)due south^2}{\sigma^2_0}
Test of equality of two variances (F-test)
F-statistic is calculated as:
Reading vii: Introduction to Linear Regression
Bones model of simple linear regression
Y = b0 + b1X + ɛ
where:
- Y is the dependent (explained) variable;
- 10 is the contained (explanatory) variable;
- b0 is the intercept;
- bi is the slope coefficient;
- ɛ is the error term.
Assumptions of simple linear regression
- Linear relationship betwixt X and Y
- Homoscedasticity (abiding variance of residuals for all observations)
- Independence between X and Y (residuals are uncorrelated for all observations)
- Normality of the residuals
Analysis of variance (ANOVA)
Sum of squares error (SSE) = the unexplained variation in Y
SSE=\sum^N_{i=1}(Y_i - \hat{Y_i})^2
Sum of squares regression (SSR) = the explained variation in Y
SSR=\sum^N_{i=1}(\chapeau{Y_i}-\overline{Y})^2
Sum of squares full (SST) = the full variation in Y = SSR + SSE
SST=\sum^N_{i=1}({Y_i}-\overline{Y})^ii
Coefficient of determination (R2) measures the % of total variation in the dependent variable that is explained by the independent variable.
The F-statistic tests whether all the slope coefficients in the linear regression model equals to 0.
F=\frac{Hateful \space Foursquare \space Regression}{Hateful \infinite Square \space Error} = \frac{MSR}{MSE}= \frac{SSR/k}{(SSE/(n-(k+1))}
Standard error of estimate (Se) in uncomplicated linear regression:
S_e=\sqrt{MSE}=\sqrt{\frac{\sum^N_{i=one}(Y_i - \hat{Y_i})^ii}{n-2}}
CFA Level 1 Quantitative Methods Tips
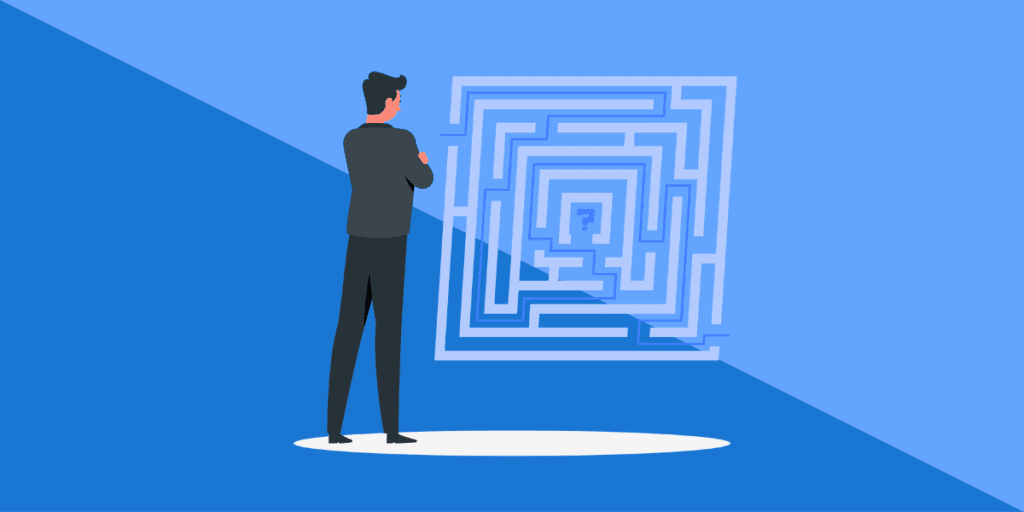
Starting time your studies (early) with Quantitative Methods
One unproblematic approach to studying for any examination is to commencement on page 1 and read through to the end.
Still, it is non uncommon for candidates to question whether to study the Ethical and Professional Standards readings offset – considering they don't neatly fit in with the residue of the curriculum. As a consequence, many recommend saving the Ethics material for terminal.
According to our best CFA Level 1 topic study lodge, it'due south a good idea to starting time with Quantitative Methods get-go, or at least early in your preparation.
These readings introduce essential topics that must exist mastered in order to be successful on exam twenty-four hour period because they are the absolute foundation of the Level 1 syllabus. Moreover, this fabric volition show up repeatedly throughout the curriculum at every level as yous progress towards your CFA lease.
Empathize the concepts, don't just memorize formulae
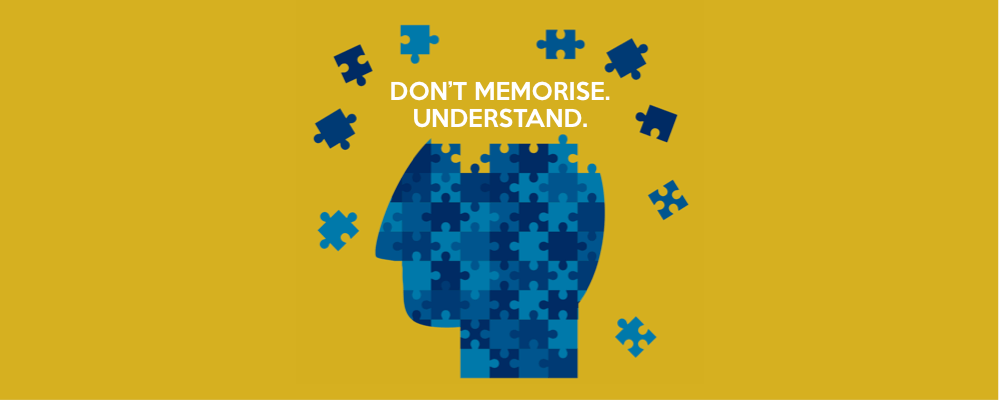
There is a natural tendency amid candidates to view the Quantitative Methods fabric every bit a long list of equations to exist memorized and worked through to produce a correct answer.
There are definitely a number of equations with which you are well-advised to go intimately familiar and you will likely give your calculator quite a workout when answering questions on this topic, but there is more to mastering this textile than number crunching.
For example, the noesis that a dataset's harmonic mean is ever less than its geometric mean, which is always less than its arithmetic mean, is but as important as memorizing the formulae used to calculate these measures.
Similarly, you don't demand to apply your estimator to know that a security's bank disbelieve yield is less than its effective annual yield.
You will come across Quantitative Methods in other topic areas
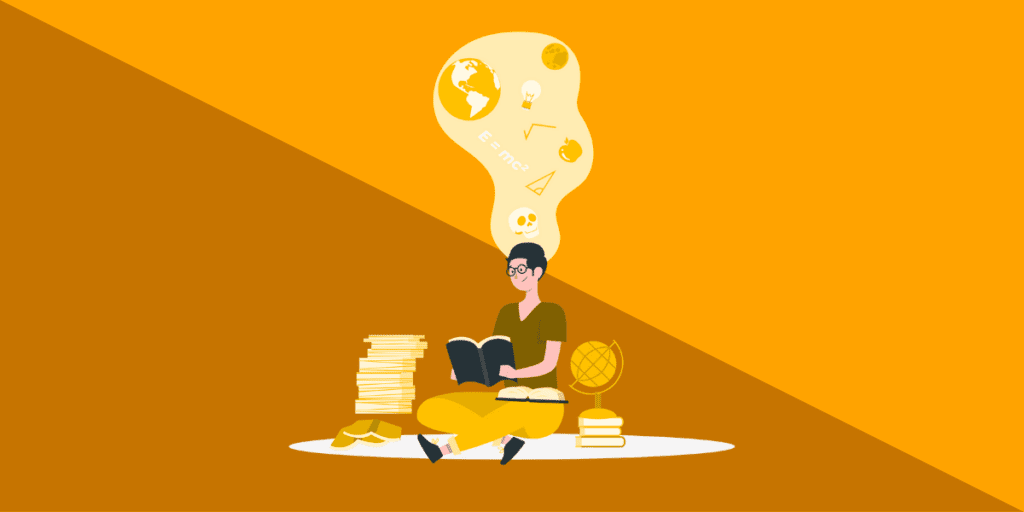
Every bit mentioned previously, Quantitative Methods topics are foundational knowledge with which you must be familiar with considering it will evidence upwards repeatedly as you progress through the curriculum.
For instance, developing a solid understanding of the yield measures presented in these readings can only benefit yous when you go to the readings on stock-still-income and corporate finance.
In notwithstanding another example, the concept of Value-at-Risk, which is covered in the Study Session on portfolio management, is outset introduced in the reading on probability distributions.
Indeed, information technology can be helpful to refer back to these readings if for no other reason than to remind yourself that you take covered these topics already and yous probably understand them better than you give yourself credit for.
More Crook Sheet articles will exist published over the coming weeks. Get ahead of other CFA candidates by signing up to our member's list to get notified.
Meanwhile, hither are other related articles that may be of interest:
- CFA Level 1 Cheat Sheets series: Economics | FRA | Corporate Issuers | Derivatives | Stock-still Income | Disinterestedness Investments | Ethics | Alt Investments | Portfolio Management
- CFA Level 1: How to Prepare and Pass CFA in 18 Months
- CFA Level ane Tips: Top 10 Advice from Previous Candidates
- 18 Actionable Means to Improve Your Study Retentivity
- How to Study Effectively: Proven Methods that Piece of work for CFA, FRM and CAIA
- The Ultimate Guide to CFA Practice Questions
Source: https://300hours.com/cfa-level-1-quantitative-methods-cheat-sheet/
Belum ada Komentar untuk "Do You Need to Know Formulas for Quant Cfa if You Use Calcultor"
Posting Komentar